Cluster Analysis: scale your customer segmentation
CA is a complex method of classifying and grouping information. Through its process, it’s possible to increase research insights and foster accurate customer segmentation.
Cluster Analysis is a statistical data processing method. It works by organizing items into groups (or clusters) by how closely associated they are with each other.
This might sound fairly simple and boring, but it takes on a totally different dimension when we talk about a great amount of data — and becomes incredibly complex and powerful to scale business insights.
Read on and find out how to use cluster analysis and research methods to foster an accurate customer segmentation.
What is Cluster Analysis?
Simply put, Cluster Analysis is a method of organizing data that seeks to group packets of information into ‘clusters’ based on how similar they are to one another. It uses data that has not been previously partitioned or organized into subsets.
CA is what we call an “unsupervised learning algorithm”, meaning that you won’t know how many clusters are present within the dataset beforehand. Because of this, Cluster Analysis is typically used when no assumptions can be made about the relationships between data points.
What it does — and what it doesn’t
This is all a bit technical, but it essentially shows you where correlations lie within the data provided. It provides you with complex associations and patterns, but it doesn’t tell you what these patterns are or what they mean.
The benefits of Cluster Analysis
Cluster Analysis is put to best use in large datasets. Smaller samples of data can usually be processed by simpler algorithms, but when it comes to massive data packs, there is no better tool for the job.
CA is also extremely useful when it comes to complex and seemingly homogeneous data sets.
If you’re analyzing information within a field you’re familiar with, you’ll probably identify a few aspects that can be pre-sorted. But CA will find you similarities even when you have no idea how to group your data points.
The biggest advantage of Cluster Analysis over other methods is its wide range of applications. If this seems strange or unusual, know that it is put to extensive use in a variety of industries, such as:
• The financial industry to discover fraudulent claims;
• In healthcare research to identify geographical locations that are associated with higher health risks;
• In marketing to produce accurate and lightning-fast customer segmentation. And that’s where we are going to focus on.
A quick recap of customer segmentation
Customer segmentation is the process of dividing your customers based on common characteristics like demographics, behaviors, lifestyle, and so on, allowing you to understand each segment in its individual entirety. This provides you with information that can be used to market to each segmented cluster of customers more effectively.
This idea of segmenting your CRM base in order to better understand the different profiles that they fall under is nothing new to the business world. Design Thinking, for example, has been taking advantage of this practice for years, under the pseudonym of Personas.
Using Cluster Analysis is an excellent way to gather insights that can be put to use in personas development — in fact, we can use CA to potentialize insights from any method of research/discovery, as we did with Jobs To Be Done within a fintech (take a look at the case at the end of the article).
Qualitative insights will always play a determinant role in the innovation process, but Cluster Analysis can provide you with a treasure trove of quantitative insights. This is an excellent example of we call a data-driven approach to market research.
RELATED CONTENT – Ebook
→ Jobs to Be Done: A Market Discovery Guide
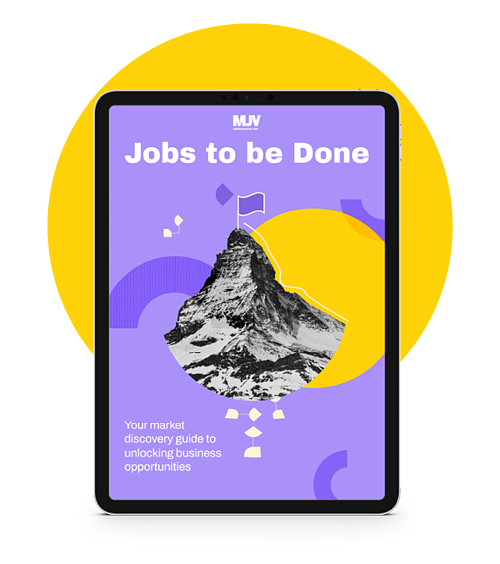
How Cluster Analysis Works?
So now you understand what Cluster Analysis is, and why it’s used, but how exactly does it work? What can we classify as a “good” Cluster Analysis? Well, for starters, Cluster Analysis is not something one does manually. It is essentially a program that will receive un-segmented data and seek to find correlations between data points within.
The possibilities of variables within a dataset is astronomical, so let’s just focus on the use of Cluster Analysis for customer segmentation.
The program sorts based on a matrix of variables, but what those variables are depends entirely on you. Regardless of whether you’re doing market segmentation or customer segmentation, there are three big things you need to keep in mind:
• Who they are
• What they do
• What they want
Cleaning Up Data & Measuring Results
So now you have your data and you’re ready to send it on through the machine, right? No. Because Cluster Analysis works with a previously unsegmented set of data, it treats each and every datapoint within the dataset equally. That means that if you input incomplete or incorrect data into the program, it will have a direct impact on the final clustering.
It’s crucial that datasets be combed for inconsistencies, errors, and gaps. Even though Cluster Analysis doesn’t require any prior data segmentation, it’s not exactly something you can use with raw, unfiltered Big Data. So how do you measure whether a Cluster Analysis did a good job or not?
There are two key metrics used when evaluating the quality of a data cluster: they’re called intracluster distance and intercluster distance.
→ Intracluster distance is the distance between the data points inside the cluster. A high-quality cluster analysis should have a small intracluster distance (this means the data within clusters are more homogenous).
→ Intercluster distance corresponds to the distance between data points in different clusters. A high-quality cluster analysis should have a large intercluster distance (this means that each individual cluster is unique amongst its neighbors, more heterogeneous).
Cluster Analysis Tools & Processes
Cluster Analysis can be put to use in a variety of different processes and in conjunction with a number of tools. While this article has focused on its use in customer segmentation & marketing research, we thought it would be good to give you a few more examples of where it can be put to use.
1. Strategic significance
When it comes to strategic planning, it can be difficult to understand the significance of a particular set of data related to a specific business question or challenge. Cluster Analysis can be used to identify significant similarities in relation to values, criteria, or factors within a data set.
2. Exploratory data analysis
Cluster Analysis can also be used within exploratory data analysis. These are essentially processes where data sets are analyzed based on the criteria of data quality and relevance to the problem. During exploratory analysis, it can be difficult to understand and extract relationships within the data set, something that CA excels at.
3. Data classification
Data classification is essentially the process of mapping existing data to find out how and where that data flows through and identifying processes that can change its content before it reaches a certain stage within it. This might sound a bit technical (and we are by no means going to go in-depth in this article), but just know that Cluster Analysis can be used to great effect within data classification.
4. Hyphotesis testing
We mentioned earlier that Cluster Analysis is best put to use within data sets that haven’t yet been segmented. When conducting research or gathering data, it’s very difficult not to develop some preconceived notions or hypotheses throughout the process. But it can also be put to use to test your hypothesis.
Raised hypotheses can be validated by running the data you used to develop them through a Cluster Analysis. The program won’t make any assumptions about the data points, so you can use it to identify non-bias similarities within the dataset. If the results of the CA match your hypothesis, then you just might be onto something, if not, it might be time to go back to the drawing board.
Cluster Analysis + Design + Market Research
Here at MJV, we believe in injecting design and marketing research into everything we do (that even includes our data-sorting algorithms). We would be remiss if we didn’t share with you some of the ways that powerful mix works within some of our projects.
Case Study: Latam Fintech Unicorn
One of the biggest fintechs in Latin America, with an swarm of followers – something previously unthinkable for the banking sector – could not classify these new clients by only income and age group. Something was missing. And we were determined to find it.
The challenge: to maintain a high level of consumer satisfaction and offer increasingly adherent financial products and services. As well as understand this new profile.
As the initial challenge was too broad, it was divided into four parts:
1. Segmentation of the population within banking services;
2. Discovery of unmet needs;
3. High-income market opportunity discovery;
4. CRM segmentation & profiling
For this, the project was structured in four different interdisciplinary segments:
• Customer & Market: Benchmarks and market research.
• Quantitative research: Customer research, data crossing, and final segmentation.
• Innovation opportunities: Using Jobs To Be Done methodology to list actions in a blue ocean strategy.
• Data Science: Data quality, exploratory data analysis, hypothesis generation, feature engineering, dashboard development, CRM base segmentation, segmentation comparison, customer base scoring, base classification automation, and segmentation documentation.
Have a taste of cluster analysis practical results. Read the full story here.
In the context of sudden changes we are all living in, it’s worth making sure you’re not missing anything important about your customers’ behaviors and needs. In this article, we’ve just explained how Cluster Analysis works scaling information through accurate customer segmentation.
The fact is that CA application possibilities are truly endless no matter the industry you might operate in. Our final piece of advice for those who have seen the value of Cluster Analysis is: don’t try this at home. You’ll need help. Are you ready for it? So hit one of our consultants — and get the staff you need to work your customer base.
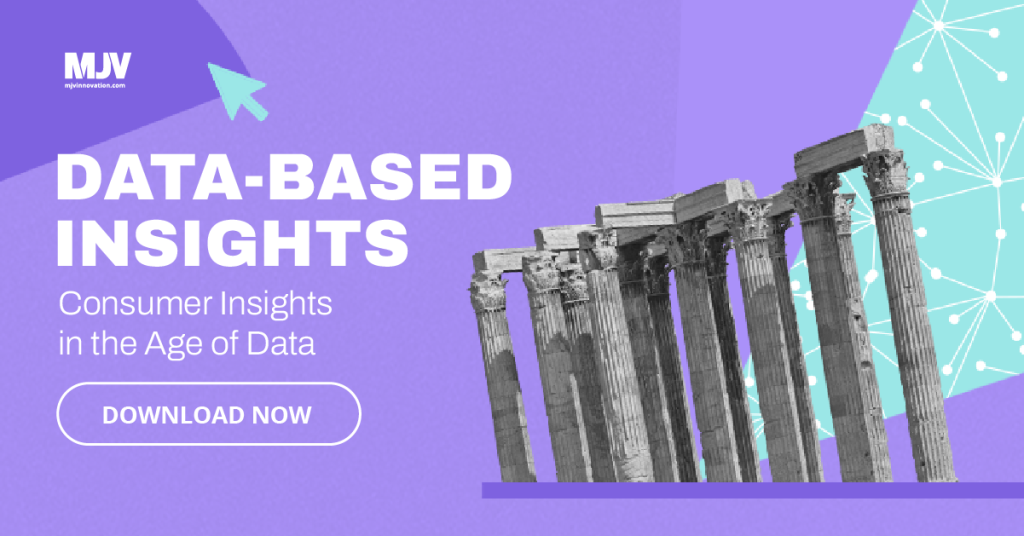